Metabolic profiling offers a snapshot of low molecular mass metabolites in biological samples, transforming our understanding of metabolic processes. It highlights the impact of microbial communities on human health, taking us a step closer towards personalized healthcare.
Using mass spectrometry in metabolic profiling
Metabolomics relies on mass spectrometry (MS), a prevalent methodology known for its high sensitivity, reproducibility, and versatility. MS is a quantitative and qualitative analytical technique that uses the mass-to-charge (m/z) ratio of previously ionized molecules to provide insights into biochemical pathways, metabolic fluxes, and the physiological status of organisms. Due to the diverse physical and chemical properties of compounds such as lipids, amino acids, and organic acids, as well as their widespread and fluctuating distribution across biological matrices, different MS-based technologies are used (Ren et al. 2018; Zhang et al. 2015).
Mass spectrometry, which consists of three main components: an ion source, a mass analyzer, and a detector. Ionization techniques such as electrospray ionization (ESI) and matrix-assisted laser desorption/ionization (MALDI) ionize molecules from solutions/solid-state, mixtures, while electron ionization (EI) is used to ionize molecules from the gas phase (after gas-chromatography (GC) separation).
Mass analyzers like time-of-flight (TOF), quadrupole, and ion trap separate ions based on their m/z ratios. Detectors such as electron multipliers and photomultiplier tubes then amplify ion signals for analysis. Liquid chromatography-mass spectrometry (LC-MS) combines liquid chromatography separation with MS detection, suitable for complex mixtures, whereas GC-MS offers high resolution for volatile compounds but requires derivatization for non-volatile ones. Each platform has advantages and limitations, guiding their selection for metabolomics studies based on the nature of the analytes and analytical requirements (Ahmad et al. 2020, Hoffmann et al. 2007).
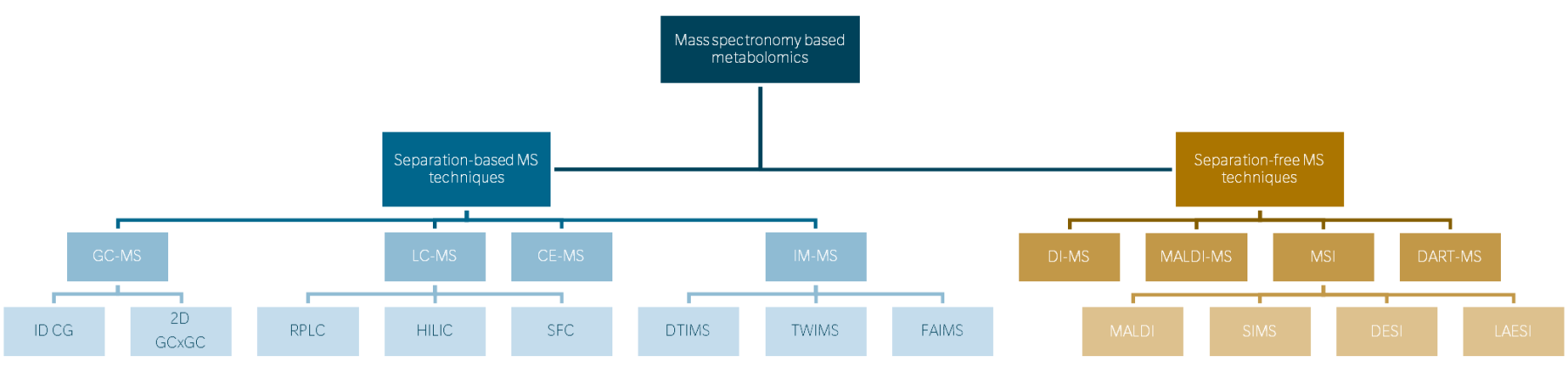
Sample preparation
Effective metabolomic analysis calls for meticulous sample preparation to extract metabolites from the biological matrices, enhance detectability, and mitigate potential interference in subsequent mass spectrometry (MS) analysis.
If you’re unsure about the optimal matrix for your study, consider referring to ‘Which sample matrix should I use for my metabolomics study?’.
While preparation techniques play a crucial role in improving the detection and stability of metabolites, they also introduce complexities and variability into the sample preparation workflow. Sample clean-up procedures are necessary to eliminate unwanted matrix components, ameliorate matrix effects and enhance the sensitivity and selectivity of MS detection (Gong et al. 2017). Despite efforts to optimize sample preparation protocols, striking a balance between maximizing metabolite detection and minimizing sample variability and matrix effects remains challenging (Fan et al. 2012).
Extraction and analysis
Metabolomics analysis relies on various extraction techniques, each tailored to specific metabolite proprieties and sample matrices. Liquid-liquid extraction (LLE), solid-phase extraction (SPE), and protein precipitation are a few examples, each offering distinct advantages and applicability across different sample types (Nováková et al. 2024).
LLE involves partitioning analytes between two immiscible liquid phases, making it ideal for extracting non-polar and moderately polar metabolites. SPE uses solid-phase sorbents to selectively retain analytes, making it applicable to a wide range of sample types with high selectivity (Schomakers et al. 2022, Chetwynd et al. 2015). By contrast, protein precipitation removes proteins using organic solvents or acids, providing a rapid and effective approach for high-throughput analyses.
Derivatization methods enhance metabolite detection and stability, particularly those with low volatility or poor ionization efficiency, but as with preparation techniques, they can complicate the sample preparation workflow (Skov et al 2015).
Data analysis and interpretation
After data preprocessing, which involves peak picking, retention time alignment, and normalization, researchers strive to mitigate systematic variations that may be lurking in the raw data. To facilitate the identification of differences between experimental conditions, the data interpretation phase uses statistical methods, which may include the following (Lamichhane et al. 2018, Cambiaghi et al. 2017):
- t-tests
- analysis of variance (ANOVA)
- linear mixed models
- multivariate analyses such as principal component analysis (PCA) and partial least-squares discriminant analysis (PLS-DA)
- metabolite set analysis such as the hypergeometric test and Fisher Exact test
- data fusion techniques such as joint and individual explained analysis (JIVE) and deeply integrated single-cell omics (DISCO).
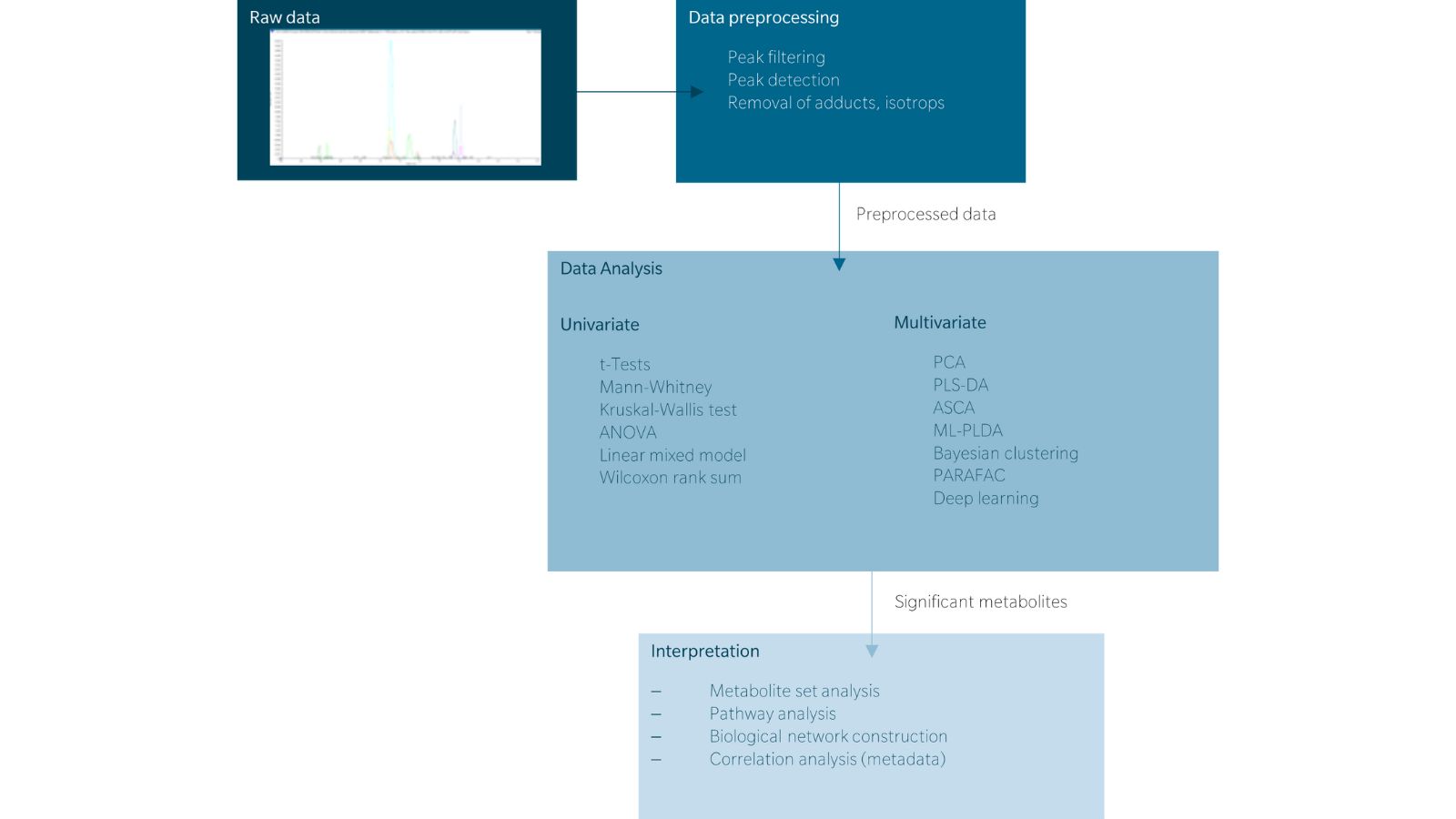
A few common challenges to look out for at this stage are handling missing values, managing high data dimensionality, and controlling false discovery rates. Researchers address these through various strategies such as imputation techniques, dimensionality reduction methods, and stringent statistical corrections. Quality control measures and validation strategies are also imperative for ensuring the robustness and reproducibility of results (Perez de Souza et al. 2024, Anwardeen et al. 2023). Multivariate data analysis techniques help find patterns and correlations within metabolomics datasets. Limonciel, 2023, introduces the “STORY Principle”, a systematic framework for interpreting metabolomics data and guiding researchers through each step.
Applications of MS-based metabolomics in recent research publications
Metabolomics can be applied in diagnostics, prognostics, biomarker discovery, treatment monitoring, and understanding disease mechanisms across various fields such as cardiometabolic diseases, diabetology, oncology, and toxicology.
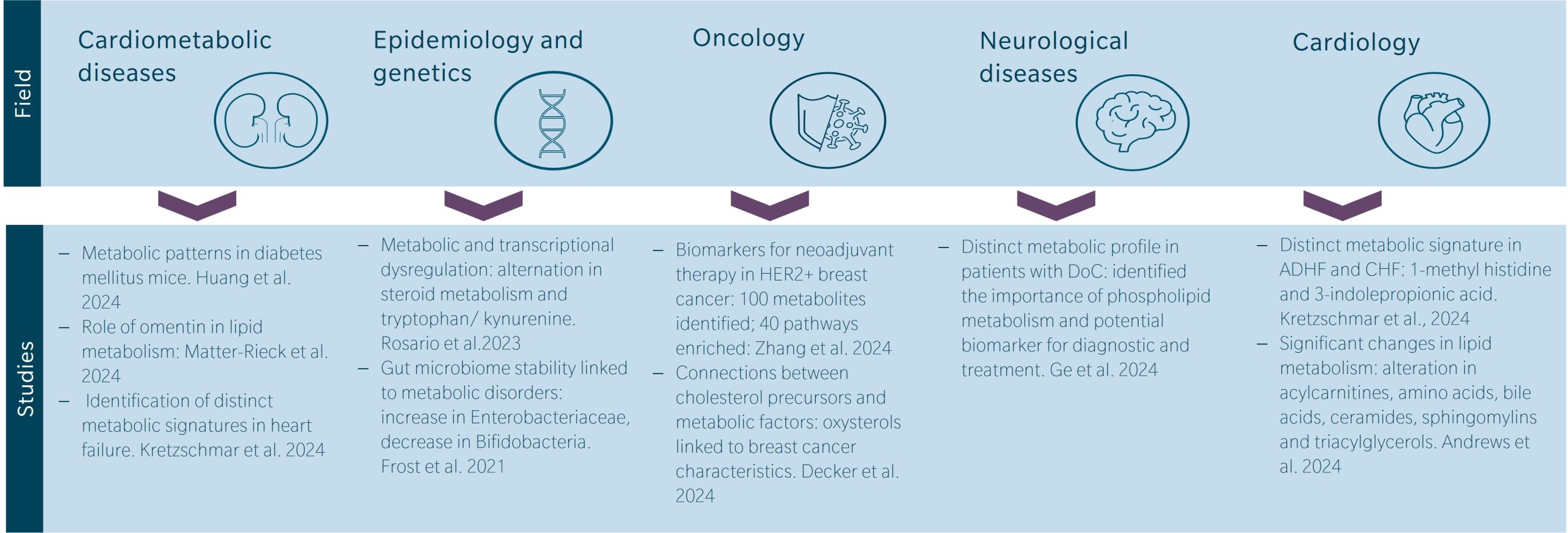
The study of cardiometabolic diseases, including diabetes, is an especially important application of MS in metabolomics research. Huang et al. 2024, investigated the protective effect of Liu-Wei-Di-Huang-Wan (a traditional Chinese formulation composed of six commonly used Chinese herbs) in mice with type 2 diabetes mellitus. They identified 30 endogenous metabolites including 3-hydroxybutyric acid, citric acid, hexadecanoic acid, and octadecanoic acid. They found that treatment with Liu-Wei-Di-Huang-Wan improved metabolic patterns in diabetic kidney disease mice, comparable to gliquidone, by regulating metabolites associated with key metabolic pathways. In another study, Matter-Rieck et al. 2024, showcase the potential role of omentin in regulating systemic metabolism, particularly lipid metabolism, particularly lipid metabolism, and influencing insulin sensitivity, further exploring its involvement in type 2 diabetes. Omentin levels were positively associated with lipids such as phosphatidylcholines (PCs) and acylcarnitines, and negatively associated with certain amino acids.
Epidemiology and genetics offer insights into disease etiology and progression. Rosario et al. 2023, identified significant metabolic and transcriptional dysregulation associated with gastrointestinal adenocarcinomas. The findings reveal alterations in pathways such as steroid metabolism and tryptophan/kynurenine metabolism, with sex-specific differences. Investigating gut microbiota stability, Frost et al. 2021, found that instability, characterized by an increase in potential pathogens like Enterobacteriaceae and a decrease in beneficial bacteria such as Bifidobacteria, was significantly associated with metabolic disorders including diabetes mellitus and fatty liver disease.
Metabolomics is also an important investigative tool in oncology, presenting the metabolic signatures linked to cancer development and treatment response. Zhang N et al. 2024, used LC-MS and GC-MS analysis of plasma metabolites to study biomarkers for neoadjuvant therapy efficacy in HER2 + breast cancer. Significant differences were found between responders and non-responders, with 100 metabolites identified and enriched in 40 pathways. Area under the curve (AUC) values for discriminating groups exceeded 0.910, and 18 metabolites showed potential for monitoring efficacy. In another study, Decker et al. 2024, described connections between cholesterol precursors, oxysterols, and diverse factors in women diagnosed with breast cancer. Their research shows the linkage of cholesterol precursors to metabolic factors like body mass index (BMI), cardiovascular disease (CVD) and oxysterols, particularly those generated via reactive oxygen species (ROS), and key characteristics of breast cancer tumors, including obesity.
Metabolic profiling is increasingly recognized in cardiology studies. A study by Kretzschmar et al. 2024, analyzed plasma metabolites in patients with acute decompensated heart failure (ADHF) and chronic heart failure (CHF). They found distinct metabolic signatures associated with each phenotype, including potential novel biomarkers for heart failure such as 1-methyl histidine and 3-indolepropionic acid. Another interesting study by Andrews et al. 2024, highlights significant changes in lipid metabolism in mice, specifically focusing on alterations in acylcarnitines, amino acids, bile acids, ceramides, sphingomyelins, and triacylglycerols. These findings pinpoint the mechanisms underlying hepatic steatosis and atherosclerosis development in mice fed the hyperhomocysteinemic diet.
Finally, in neuroscience, Ge et al. 2024, conducted a metabolomic analysis from 48 patients with disorders of consciousness (DoC), showing distinct metabolic profiles linked to etiology, consciousness levels, and prognosis. Their findings highlight the critical role of phospholipid metabolism and identify potential biomarkers for improved diagnosis and treatment of DoC.
As mass spectrometry evolves, we’ll see more opportunities to apply the methodology within metabolomics studies. If you are considering using this technique in your own study, feel free to contact the biocrates team with any questions.
References
Andrews, S.G. et al.: Diet-Induced Severe Hyperhomocysteinemia Promotes Atherosclerosis Progression and Dysregulates the Plasma Metabolome in Apolipoprotein-E-Deficient Mice (2024) Nutrients | DOI: 10.3390/nu16030330
Anwardeen, N.R.et al.: Statistical methods and resources for biomarker discovery using metabolomics (2023) BMC Bioinformatics 24 | DOI:10.1186/s12859-023-05383-0
Cambiaghi A et al.: Analysis of metabolomic data: tools, current strategies and future challenges for omics data integration (2017) Briefings in Bioinformatics | DOI: 10.1093/bib/bbw031
Causon J.: Review of sample preparation strategies for MS-based metabolomic studies in industrial biotechnology (2016) Analytica Chimica Acta | DOI: 10.1016/j.aca.2016.07.033
Chetwynd J. et al.: Solid-Phase Extraction and Nanoflow Liquid Chromatography-Nanoelectrospray Ionization Mass Spectrometry for Improved Global Urine Metabolomics (2014) Analytical Chemistry | DOI: doi.org/10.1021/ac503769q
Decker, N.S et al.: Associations between lifestyle, health, and clinical characteristics and circulating oxysterols and cholesterol precursors in women diagnosed with breast cancer: a cross-sectional study. (2024) Sci Rep 14 | DOI: 10.1038/s41598-024-55316-x
Fan, T.WM.: Considerations of Sample Preparation for Metabolomics Investigation. (2012) The Handbook of Metabolomics. Methods in Pharmacology and Toxicology | DOI: 10.1007/978-1-61779-618-0_2
Frost F. et al.: Long-term instability of the intestinal microbiome is associated with metabolic liver disease, low microbiota diversity, diabetes mellitus and impaired exocrine pancreatic function (2021) Gut | DOI: 10.1136/gutjnl-2020-322753
Ge Q, et al.: Serum metabolism alteration behind different etiology, diagnosis, and prognosis of disorders of consciousness (2024) Chin Neurosurg J. | DOI: 10.1186/s41016-024-00365-4
Gong Z-G. et al.: The Recent Developments in Sample Preparation for Mass Spectrometry-Based Metabolomics (2017) Critical Reviews in Analytical Chemistry, 47(4), 325–331 | DOI: 10.1080/10408347.2017.1289836
Hoffmann E. et al.: Mass Spectrometry: Principles and Applications (2007) Hoboken, NJ: John Wiley & Sons. | ISBN: 978-0-470-03310-4
Huang JH. et al.: A GC-MS-Based Metabolomics Investigation of the Protective Effect of Liu-Wei-Di-Huang-Wan in Type 2 Diabetes Mellitus Mice (2020) Int J Anal Chem. Aug | DOI: 10.1155/2020/1306439
Javed A et al.: Basic Principles and Fundamental Aspects of Mass Spectrometry (2022) Mass Spectrometry in Food Analysis | DOI: 10.1201/9781003091226-2
Kretzschmar T. et al.: Metabolic Profiling Identifies 1-MetHis and 3-IPA as Potential Diagnostic Biomarkers for Patients With Acute and Chronic Heart Failure With Reduced Ejection Fraction. (2024) Circ Heart Fail | DOI: 10.1161/CIRCHEARTFAILURE.123.010813
Lamichhane S.:Chapter Fourteen – An Overview of Metabolomics Data Analysis: Current Tools and Future Perspectives (2018) Comprehensive Analytical Chemistry | DOI: 10.1016/bs.coac.2018.07.001
Limonciel A. The STORY principle A guide to the biological interpretation of metabolomics (2023) biocrates life sciences ag | DOI: 10.1016/bs.coac.2018.07.001
Nováková S. et al.: Comparison of Various Extraction Approaches for Optimized Preparation of Intracellular Metabolites from Human Mesenchymal Stem Cells and Fibroblasts for NMR-Based Study (2024) Metabolites | DOI: 10.3390/metabo14050268
Perez de Souza L. et al.: Computational methods for processing and interpreting mass spectrometry-based metabolomics. (2024) Essays Biochem | DOI: 10.1042/EBC20230019
Ratter-Rieck J.: Omentin associates with serum metabolite profiles indicating lower diabetes risk: KORA F4 Study (2024) BMJ Open Diabetes Research and Care | DOI: 10.1136/bmjdrc-2023-003865
Ren J-L. et al.: Advances in mass spectrometry-based metabolomics for investigation of metabolites (2018) Royal Society of Chemistry | DOI: 10.1039/C8RA01574K
Rosario D. et al.: Systematic analysis of gut microbiome reveals the role of bacterial folate and homocysteine metabolism in Parkinson’s disease (2021) Cell Reports 34 | DOI: 10.1016/j.celrep.2021.108807
Schomakers V. Et al.: Polar metabolomics in human muscle biopsies using a liquid-liquid extraction and full-scan LC-MS (2022) TAR Protocols | DOI: 10.1016/j.xpro.2022.101302
Skov K. et al.: LC–MS analysis of the plasma metabolome—A novel sample preparation strategy (2015) Journal of Chromatography B | DOI: 10.1016/j.jchromb.2014.11.033
Zhang A. et al.: Metabolomics for Biomarker Discovery: Moving to the Clinic (2015) Biomed Res Int. | DOI: 10.1155/2015/354671
Zhang N et al.: Metabolomics assisted by transcriptomics analysis to reveal metabolic characteristics and potential biomarkers associated with treatment response of neoadjuvant therapy with TCbHP regimen in HER2 + breast cancer. (2024) Breast Cancer Res. | DOI: 10.1186/s13058-024-01813