A new era in consumer health monitoring
Lifestyle and diet are widely recognized as significant determinants of health, driving demand for personalized health solutions (Rafiq et al. 2021). As individuals look for ways to actively manage their well-being, consumer tests such as microbiome sequencing for gut health or proteomics-based longevity assessments are gaining traction. These tests often combine biological measurements with self-reported dietary questionnaires to deliver tailored diet recommendations (Brennan 2017; Guasch-Ferré et al. 2018; Rafiq et al. 2021).
However, self-reported data is prone to inaccuracies, as consumers frequently misestimate their intake of vegetables, red meat, and other foods (Brennan 2017). Scientific research highlights the limitations of self-reported dietary data, with error rates for caloric intake and food portion size ranging from 30% to 88% (Rafiq et al. 2021). These misestimations stem from factors such as memory bias, cultural differences, and the inherent complexity of assessing habitual diets (Gibson et al. 2017).
Analyzing metabolites in biological samples, such as blood, urine, or saliva, can address these challenges. Metabolomics provides a snapshot of an individual’s current nutritional and physiological state, offering a robust, unbiased alternative to complement and validate traditional questionnaires (Gibson et al. 2017; Brennan 2017; Guasch-Ferré et al. 2018). By bridging the gap between subjective data and objective biomarkers, metabolomics enables more accurate diet assessments and personalized nutrition recommendations. This integrated approach deepens our understanding of the dietary impacts on health, facilitating more effective lifestyle interventions aimed at disease prevention.
How dietary patterns shape health through metabolic profiles
Metabolomics research shows that dietary intake is better reflected through food group biomarkers than isolated nutrients. Synergistic interactions between dietary components influence the metabolic response, and metabolomics captures these complex relationships. Studies consistently identify metabolite signatures linked to various food groups, including fruits, vegetables, high-fiber grains, meats, seafood, legumes, nuts, dairy, and caffeinated beverages (Rafiq et al. 2021).
For example, betaine and betaine-related metabolites are associated with fruits and vegetables, with proline betaine linked to citrus fruit consumption and tryptophan betaine to legume consumption (Pekkinen et al. 2015; Lever et al. 2010). High-fiber diets contribute to the production of short-chain fatty acids (SCFAs) by gut microbiota, essential for maintaining gut health and metabolic regulation (Nogal et al. 2021). Meats and seafood provide amino acids and carnitines, along with metabolites such as trimethylamine N-oxide (TMAO), a marker linked to cardiovascular risk (Gatarek et al. 2021; Wang et al. 2022). Fish intake is reflected by the concentrations of omega-3 fatty acids, with eicosapentaenoic acid (EPA) and docosahexaenoic acid (DHA) being the most reliable indicators. The vitamin B3-related trigonelline is considered a biomarker of coffee intake due to its high concentrations in coffee and related products (Mohamadi et al. 2018).
Metabolic profiling of these food groups helps identify dietary patterns that align with either protective or detrimental health outcomes:
- Risk-associated diets
Diets high in processed meats, sugary foods, and refined grains – characteristic of a typical Western diet – are associated with obesity, metabolic disorders, cardiovascular disease, cancer and inflammation (Clemente-Suárez et al. 2023).
- Protective diets
Diets rich in vegetables, whole grains, and fish correlate with metabolomic profiles characterized by beneficial metabolites like betaines, omega-3 fatty acids, and short-chain fatty acids (SCFAs) (Emwas et al. 2021; Nogal et al. 2021; Pekkinen et al. 2015; Rafiq et al. 2021). Two typical examples are the Mediterranean and Nordic diets, which differ primarily in their preferred oils: extra virgin olive oil in the Mediterranean, and rapeseed oil in Nordic countries, which contains oleic acid, linoleic acid and alpha-linolenic acid. The Nordic diet emphasizes rye, barley, and oats as staple whole grains and favors berries over other fruit.
While specific metabolite markers can be used to provide insights into dietary habits, such as naringenin as a biomarker for grapefruit intake, they do not necessarily reflect overall dietary quality or health outcomes. In the context of disease prevention and nutritional research, it is more meaningful to assess whether an individual follows a healthy dietary pattern as a whole, rather than pinpointing the exact source of nutrients. Whether vitamins come from a grapefruit or a kiwi is ultimately less important than ensuring a diet is nutrient-rich, diverse, and aligned with established health guidelines.
To objectively assess diet quality, standardized scoring systems known as diet quality indices have been developed. These evaluate overall dietary patterns based on adherence to established nutritional guidelines, incorporating both nutrient density and food group composition. They are widely used in epidemiological studies, public health assessments, and clinical research toanalyze the impact of diet on chronic diseases, longevity, and overall health. A large cohort study by Kim et al. identified 17 metabolites that were significantly associated with better diet scores across four major healthy dietary indices (Healthy Eating Index, Alternative Healthy Eating Index, Dietary Approaches to Stop Hypertension and alternate Mediterranean diet). The study shows how metabolite concentrations directly reflect dietary habits because the molecules taken up with the diet feed into the universal core metabolic pathways (Kim et al. 2021). Furthermore, these metabolites might serve as biomarkers for healthy dietary patterns, providing an objective way to measure diet quality and its impact on health.
The science of well-being – how diet and stress influence metabolic health
In addition to reflecting dietary patterns, certain metabolites and their ratios serve as biomarkers for specific conditions and diseases, providing valuable insights into overall well-being and metabolic health.
Betaine and choline are obtained from the diet or synthesized de novo, with choline serving as a precursor for betaine. Both are vital methyl-donor nutrients involved in lipid metabolism, liver function, and the methylation cycle (Chang et al. 2022), and their uptake and balance are critical for metabolic health. While adequate choline intake supports lipid transport and hepatic function (Chang et al. 2022), excess serum choline is associated with metabolic syndrome risk factors such as dyslipidemia, hyperglycemia and cardiovascular disease. The increased risk for cardiovascular disease is linked to the microbiome: some gut bacteria convert choline (and sometimes betaine) to trimethylamine, which is taken up by the human host and further oxidized to TMAO. Trimethylamine and TMAO are both known risk factors for cardiovascular disease (Jang et al. 2021). In contrast, higher betaine levels correlate with favorable lipid and glycemic profiles (Gao et al. 2019). Consequently, optimal dietary intake of choline and betaine significantly reduces the risk of hepatic steatosis and improves indicators of metabolic syndrome (Gao et al. 2019; Chang et al. 2022).
A nutritional study with more than 10,000 participants in Spain found a correlation between eating habits and mood. A diet including fruit, nuts, legumes, and a high ratio of monounsaturated to saturated fats associated with better mood and lower risk of depression (Sánchez-Villegas et al. 2009).
Beyond diet, lifestyle factors — especially stress — are key factors affecting overall well-being. Sustained stress can elevate circulating cortisol levels, contributing to allostatic load, a state where chronic physiological strain disrupts the body’s regulatory networks. High cortisol levels have been linked to mood disorders, anxiety, sleep disturbances, and metabolic imbalances (Rodriquez et al. 2019).
Quality sleep is crucial for stress management, with γ-aminobutyric acid (GABA) playing a key role as a neurotransmitter known for its calming effects. Low GABA levels correlate with reduced sleep quality, though human trial data on the benefits of GABA supplementation remain inconclusive. The microbiome is also a major GABA producer. More research with standardized methodologies is needed to determine the interplay between stress, sleep, and metabolism, and the efficacy of nutritional interventions for stress resilience and metabolic health (Hepsomali et al. 2020).
Metabotyping – tailored health solutions
Metabotyping identifies metabolic phenotypes based on a wide range of factors, including diet, anthropometric measures, clinical parameters, metabolomics data, and the gut microbiota (Hillesheim et al. 2020). This comprehensive approach gains further depth by integrating metabolomics with other omics technologies – such as genomics, transcriptomics, and proteomics – as well as fitness tracking devices. Wearable technologies that monitor physical activity, heart rate variability, and sleep patterns provide valuable complementary insights (Kelly et al. 2020).
This comprehensive approach can be used to provide customized dietary guidance. For instance, individuals with similar metabotypes may share common metabolic responses to specific foods or nutrients, enabling interventions that are highly targeted and effective. Furthermore, metabotyping can identify individuals at higher risk for metabolic diseases like type 2 diabetes or cardiovascular disorders by analyzing biomarkers such as glucose tolerance, lipid profiles, and inflammatory markers (Palmnäs et al. 2020).
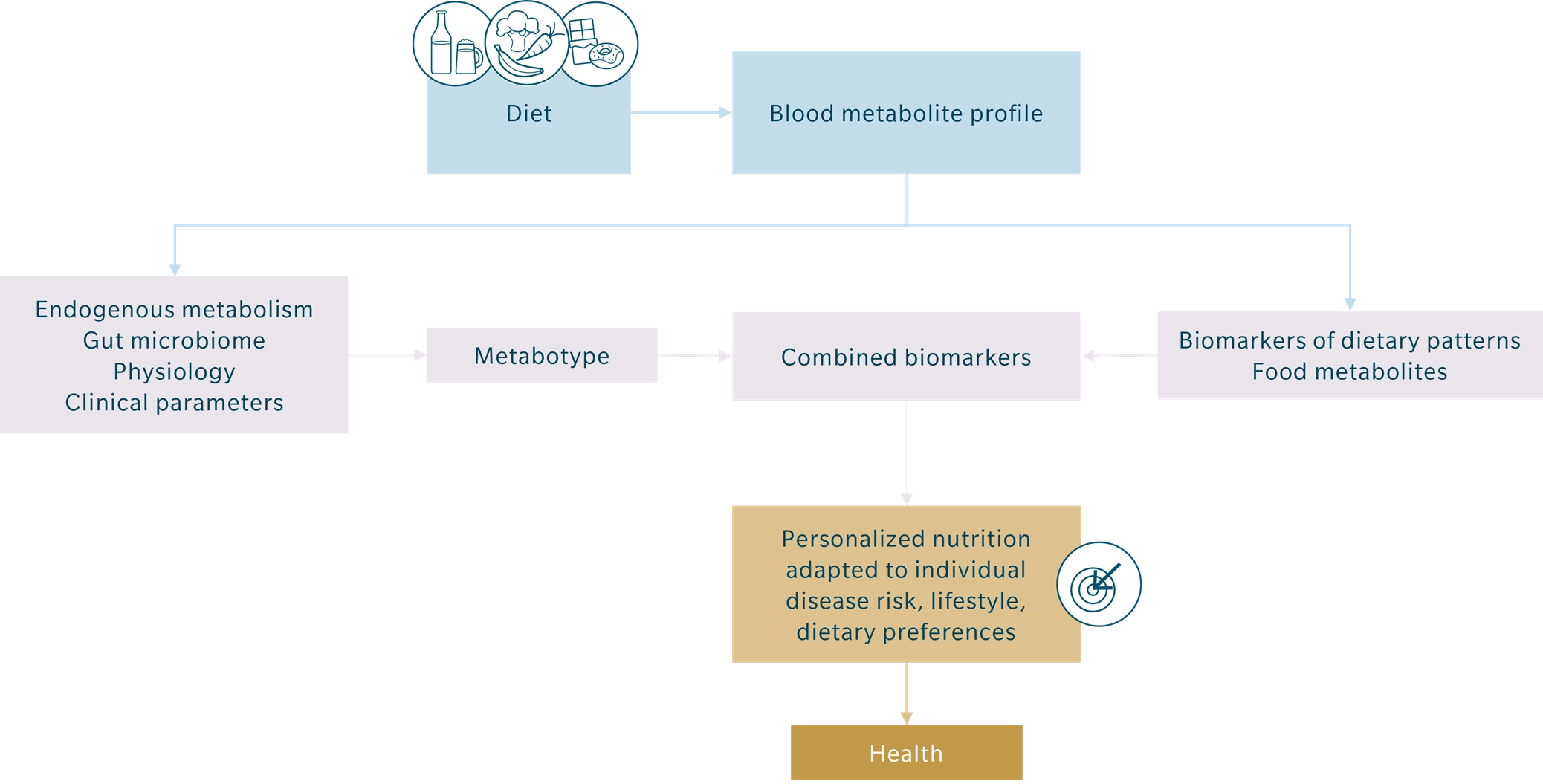
Figure 1: Personalized nutrition using metabolomics
Interestingly, research on personalized nutrition has uncovered significant individual variability in metabolic responses to identical foods. Studies have shown that, even when consuming the same meals, individuals display highly variable postprandial glucose responses, shaped by their distinct metabolic and microbiome profiles. While some experience sharp glucose spikes, others exhibit minimal increases, emphasizing the crucial role of gut microbiome composition and metabolic phenotypes in glycemic regulation (Zeevi et al. 2015).
Building on this, further research has shown that individuals in different metabotype subgroups exhibit varying glucose responses to an oral glucose tolerance test. Those classified in “intermediate” and “unfavorable” metabotypes tend to have significantly higher postprandial glucose concentrations, with the unfavorable subgroup displaying the highest glycemic response (Dahal et al. 2022).
Additionally, dietary fiber interventions reveal differential metabolic benefits depending on metabotype. While a 12-week fiber intervention led to modest reductions in metabolic risk factors overall, individuals with poorer baseline metabolic health experienced the greatest improvements in insulin levels, cholesterol, and blood pressure. These findings suggest that targeted dietary interventions may be particularly beneficial for those with higher metabolic risk (Dahal et al. 2022).
Dried blood spot sampling innovations – analytics with the donor in mind
One challenge of incorporating metabolomics into consumer health testing is the logistics of sample collection and transport. A scalable approach relies on accessible samples. Plasma samples, though widely used in research, require professional blood draws, rapid processing, and cold-chain logistics to preserve sample integrity. These requirements are impractical for at-home consumer tests.
Dried blood sampling addresses these limitations by offering a simple, reliable alternative for metabolite analysis. Dried blood sampling involves collecting small volumes of blood through a finger-prick, which are then dried on specialized devices. These samples are stable at ambient temperatures, eliminating the need for refrigeration or expedited transport.
Innovative dried blood sampling methods include volumetric absorptive microsampling (VAMS) devices such as the widely tested Mitra® tips, and newer technologies like the capillary-based qDBS Capitainer® and the TASSO-M20™ devices, which eliminate the need for a finger prick (Couacault et al. 2024). These technologies facilitate the straightforward collection of precise and predefined blood volumes, even by untrained individuals.
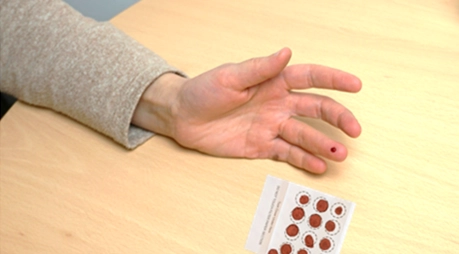
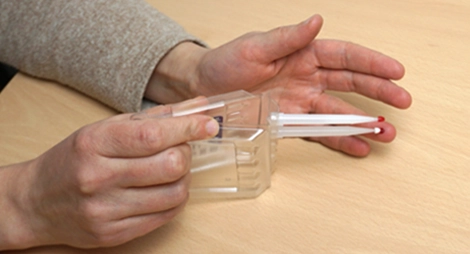
Figure 2: At home sampling with classical DBS cards vs. Mitra® devices as a volumetric alternative
Metabolomic studies confirm the reliability of dried blood in measuring key biomarkers of nutrition and wellbeing (Protti et al. 2022; Kok et al. 2019). Metabolites like vitamin D, SCFAs, and amino acids remain stable in dried blood samples, ensuring accurate quantification. A key advantage of VAMS is its ability to preserve the stability of small molecules, which are particularly informative for assessing an individual’s health (Qiu et al. 2023).
Dried blood sampling offers a scalable solution for integrating advanced metabolomics into precision nutrition, making personalized health insights more accessible to a broader audience. By combining user-friendly devices and robust analytical capabilities, this method has the potential to revolutionize precision health monitoring.
Companies offering consumer health tests have begun to implement these new technologies into their product line. For example, the medical diagnostic laboratory biovis, operating mainly in central Europe via medical practitioners, has recently launched the Prevent 360 test analyzing more than 70 metabolites from dried blood Mitra devices to provide comprehensive insight into the patient‘s health and well-being. Considering the advantages of combining these sampling devices with metabolomics, more companies are bound to follow their example.
Advancing consumer testing options with metabolomics tools
Metabolomic analysis of dried blood samples requires robust absolute quantification of metabolites of different classes in a single run. Ideally, this should be standardized so local laboratories can perform measurements in different countries. The biocrates SMartIDQ alpha kit represents a major step forward in this regard. Specifically optimized for high throughput use with dried blood samples, this kit measures a comprehensive panel of health-related metabolites, enabling detailed nutritional and metabolic assessments.
For interpretation, it is important to consider not only the single metabolite concentrations, but also their sums and ratios. which help extract the biological significance of individual differences, linking metabolomics data to biological pathways and enzyme activities.
In conditions such as hepatic encephalopathy and liver cirrhosis, the Fischer ratio decreases due to impaired liver function. This occurs as AAAs accumulate since the liver is the only organ that catabolizes them, while BCAAs are preferentially used in the muscles for energy metabolism. The progressive decline reflects worsening liver function and metabolic imbalance (Fischer et al. 1975; Soeters et al. 1976).
The MetaboINDICATOR tool that accompanies biocrates kit software provides such advanced data interpretation by calculating more than 120 predefined sums and ratios of metabolite concentrations. The combination of cutting-edge technology and advanced tools enables laboratories and healthcare providers to deliver meaningful, personalized insights to consumers, combining state-of-the-art science and practical applications in precision health.
Empowering health monitoring through metabolomics
Predictive insights from metabolomics can identify early markers of disease risk, enabling timely interventions and fostering a proactive approach to health management. Personalized recommendations, based on robust metabolomic data, empower individuals to make informed dietary and lifestyle changes tailored to their unique health profiles. This preventive approach significantly reduces the burden of chronic disease, supporting public health objectives and aligning with integrative medicine’s focus on addressing the root causes of illness together with individualized treatment. The SMartIDQ alpha kit exemplifies how metabolomics can bring the integrative medicine approach into everyday life. Metabolomics is central to the future of 5P medicine – predictive, personalized, preventive, population-based, and participatory –advancing both nutrition science and consumer health solutions.
References
Brennan, L.: Metabolomics: a tool to aid dietary assessment in nutrition (2017) Current Opinion in Food Science | 10.1016/j.cofs.2017.09.003
Chang, T.-Y. et al.: Optimal Dietary Intake Composition of Choline and Betaine Is Associated with Minimized Visceral Obesity-Related Hepatic Steatosis in a Case-Control Study (2022) Nutrients | 10.3390/nu14020261
Clemente-Suárez, V. J. et al.: Global Impacts of Western Diet and Its Effects on Metabolism and Health: A Narrative Review (2023) Nutrients | 10.3390/nu15122749
Couacault, P. et al.: Targeted and Untargeted Metabolomics and Lipidomics in Dried Blood Microsampling: Recent Applications and Perspectives (2024) Analytical Science Advances | 10.1002/ansa.202400002
Dahal, C. et al.: Evaluation of the Metabotype Concept After Intervention with Oral Glucose Tolerance Test and Dietary Fiber-Enriched Food: An Enable Study (2022) NMCD | 10.1016/j.numecd.2022.06.007
Emwas, A.-H. M. et al.: You Are What You Eat: Application of Metabolomics Approaches to Advance Nutrition Research (2021) Foods | 10.3390/foods10061249
Fischer, J. E. et al.: The Role of Plasma Amino Acids in Hepatic Encephalopathy (1975) Surgery | 10.1016/0002-9610(74)90009-9
Gao, X. et al.: Low Serum Choline and High Serum Betaine Levels Are Associated with Favorable Components of Metabolic Syndrome in Newfoundland Population (2019) Journal of Diabetes and Its Complications | 10.1016/j.jdiacomp.2019.06.003
Gatarek, P. et al.: Trimethylamine N-Oxide (TMAO) in Human Health (2021) EXCLI Journal | 10.17179/excli2020-3239
Gibson, R. S. et al.: Measurement Errors in Dietary Assessment Using Self-Reported 24-Hour Recalls in Low-Income Countries and Strategies for Their Prevention (2017) Advances in Nutrition | 10.3945/an.117.016980
Guasch-Ferré, M. et al.: Use of Metabolomics in Improving Assessment of Dietary Intake (2018) Clinical Chemistry | 10.1373/clinchem.2017.272344
Hepsomali, P. et al.: Effects of Oral Gamma-Aminobutyric Acid (GABA) Administration on Stress and Sleep in Humans: A Systematic Review (2020) Frontiers in Neuroscience | 10.3389/fnins.2020.00923
Hillesheim, E. et al.: Metabotyping and Its Role in Nutrition Research (2020) Nutrition Research Reviews | 10.1017/S0954422419000179
Jang, H. et al.: Changes in Plasma Choline and the Betaine-to-Choline Ratio in Response to 6-Month Lifestyle Intervention (2021) Nutrients | 10.3390/nu13114006
Kelly, R. S. et al.: Metabolomics, Physical Activity, Exercise and Health: A Review of the Current Evidence (2020) Biochimica et Biophysica Acta | 10.1016/j.bbadis.2020.165936
Kim, H. et al.: Serum Metabolites Associated with Healthy Diets in African Americans and European Americans (2021) The Journal of Nutrition | 10.1093/jn/nxaa338
Rafiq, T. et al.: Nutritional Metabolomics and the Classification of Dietary Biomarker Candidates: A Critical Review (2021) Advances in Nutrition | 10.1093/advances/nmab054
Rodriguez, E. J. et al.: Allostatic Load: Importance, Markers, and Score Determination in Minority and Disparity Populations (2019) Journal of Urban Health | 10.1007/s11524-019-00345-5
Wang, Z. et al.: Circulating Trimethylamine N-Oxide Levels Following Fish or Seafood Consumption (2022) European Journal of Nutrition | 10.1007/s00394-022-02803-4
Zeevi, D. et al.: Personalized Nutrition by Prediction of Glycemic Responses (2015) Cell | 10.1016/j.cell.2015.11.001