mGWAS to enhance genomic analysis results with metabolomics
Genome-wide association studies (GWAS) investigate genomic variants (single-nucleotide polymorphisms (SNPs)) across different individuals to identify associations with a specific trait or phenotype. Over the past 15 years, GWAS have uncovered many variants associated with complex traits, but identifying the causal gene(s) is still a major challenge. More recently, integrating the GWAS dataset with other omics data has emerged as a promising approach to identify these causal genes (Mountjoy, E. et al. 2021).
Identifying causal genes for genetic diseases is not only of academic interest but pivotal for the development of therapeutic approaches. Traditional drug development is a knowledge-based approach that starts with a hypothesis based on limited data. The hypothesis is then put to the test in cell lines to qualify a potential target, before moving to preclinical research in animal models that only moderately represent human disease. More than 90% of all drug candidates fail in the preclinical stage (Sun et al. 2022), mostly because of safety concerns or lack of efficacy. In contrast, precision medicine uses a human-centric, hypothesis-free approach to drug discovery grounded in real-world data, using multiomics datasets from large populations evaluated with deep learning for target relevance and causality. Switching to this approach increased the success rate of Astra Zeneca’s drug development (from drug candidate to approval) from 4% to 19% (Morgan et al. 2018).
Why metabolomics?
Combining GWAS with metabolomics (mGWAS) shows particular promise. Metabolomics is used to analyze pathophysiological processes and map metabolites to biochemical pathways. When combined with GWAS datasets, metabolite profiling helps establish functional links to genes associated with clinical disease phenotypes. This way, mGWAS identifies genetically influenced metabotypes that correspond to phenotype-converting genetic variations. mGWAS qualifies genetic associations by effect size — indicating the phenotype-converting potential — and thus reveals potential drug targets for drug development.
Including metabolomics has an advantage over other omics in GWAS due to a marked p-value gain when using metabolite ratios. Metabolite ratios represent the flux through a biochemical pathway when a pair of metabolites is connected. For example, the ratio of an enzymatic reaction product to the source metabolite characterizes enzyme activity much better than either metabolite concentration alone. This comes with statistical benefits:
- Metabolite ratios increase the statistical power by reducing the overall biological variability.
- They reduce the impact of systematic experimental errors.
The p-gain statistic is a measure for whether a ratio between two metabolite concentrations carries more information than the two corresponding metabolite concentrations alone (Petersen et al. 2012). When this is the case, and metabolite concentrations are affected by the gene in question, including metabolite ratios in the GWAS analysis will lead to markedly lower p-values, highlighting the relevant associations.
Including metabolite sums instead of single metabolites can also make sense when studying lipids, as lipid-converting enzymes usually process several lipids with similar configuration such as similar fatty acid side chain lengths. The biocrates MetaboINDICATOR™ is a useful tool exploring metabolite sums and ratios relevant to health and disease.
Discovery of potential drug targets with mGWAS
Montasser and colleagues published a study on cardiovascular diseases that illustrates the potential of mGWAS (Montasser et al. 2022). They performed a GWAS on a discovery cohort of 650 individuals from the Old Order Amish founder population. The chance of identifying previously unknown disease associations is elevated in founder populations because certain variants are enriched to a higher frequency due to genetic drift. They discovered about eight million genetic variants.
Trying to identify the relevant ones is like looking for a needle in a haystack. To narrow down the results, they also performed lipidomics and integrated their concentration results on 355 lipid species from 14 different classes with their GWAS outcomes. This mGWAS analysis resulted in 12 significant associations. Seven of these were already known, and the five remaining significant associations represented novel associations between SNPs and cardio protection, cholecystitis, atherosclerosis, blood pressure, and inflammation, respectively. Integrating metabolomics in their GWAS thus resulted in five novel potential drug targets instead of hundreds of associations with uncertain relevance.
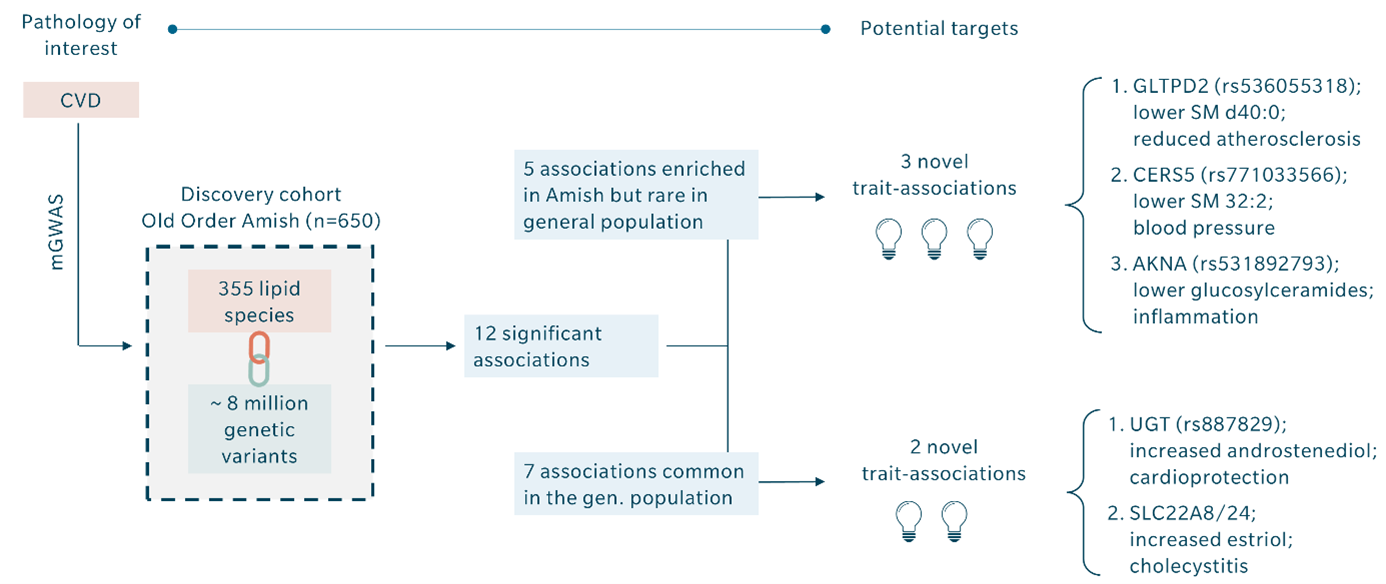
Naturally, the chances for identification of relevant variants increase with the number of metabolites covered. For example, metabolomics results obtained with the MxP Quant 500 XL kit, which covers up to 1,019 metabolites from 39 biochemical classes, bear a higher likelihood of success than a smaller kit or assay.
From weak associations to causality
The full power of mGWAS is realized when combined with Mendelian randomization. Mendelian randomization uses the measured variation in candidate genes to assess the causal effect a gene variant has on a disease by affecting metabolite concentrations or ratios, diminishing the need for an additional randomized controlled validation study. Mendelian randomization assumes that genetic variants are:
- Associated with the disease
- Not related to confounders
- Not associated with the disease through an alternative pathway in which the metabolite is not involved.
The causal effect is calculated as the variable ß̂ for each association:
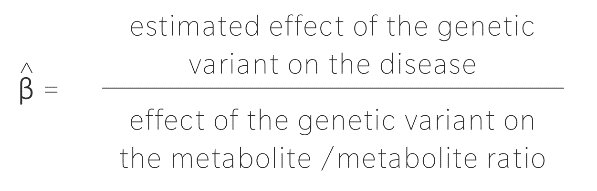
Significant results in Mendelian randomization mean that a metabolite or a metabolite ratio is related to a specific disease via a specific genetic variant (Davies et al. 2018). Thus, that variant may become a target for that disease.
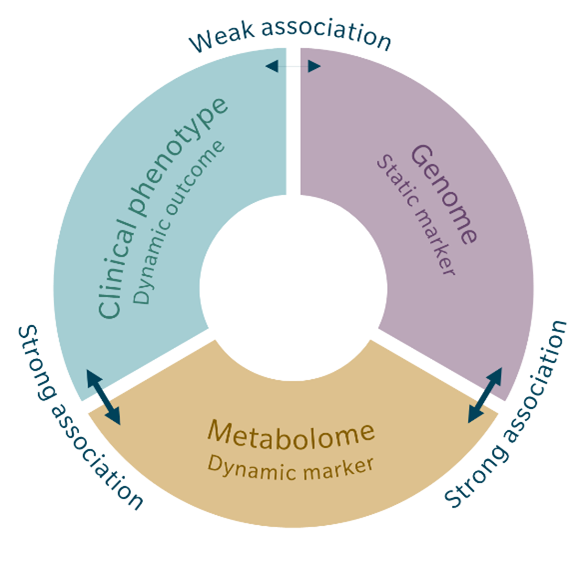
In other words, Mendelian randomization refines mGWAS data by testing for causality. The direct link between a disease or other phenotype and the corresponding genetic variant is usually quite weak, due to environmental influences. However, metabolites act as an intermediate phenotype involved in the development of the disease. The link between genetic variant and metabolite concentration is usually stronger than the association between genetic variant and disease because the relationship is more direct and independent of environmental exposures. The strength of an association is further increased when using metabolite ratios.
The same is true for the association of a certain metabolite concentration or metabolite ratio with a certain phenotype. Mendelian randomization uses the strong association between genetic variant and metabolite and the strong association between metabolome and clinical phenotype instead of the weak association between genetic variant and clinical phenotype to calculate causality. Because the environmental factors play a smaller role for these associations, they can be ignored for the causality calculation (Genetics meets metabolomics 2012). This also minimizes the risk of reverse causation, where the disease itself might appear to cause the differences in metabolite concentrations (Bowden et al. 2019; Le Chang et al. 2023).
A few examples for mGWAS studies combined with Mendelian randomization are provided in the following table:
Phenotype | Metabolite | Gene (Genetic variant) | Reference |
Gallstone risk | Campesterol ↓ | ABCG8 (rs6544713) | Yin et al. 2022 |
Arterial hypertension (HTA) | Acetoacetate ↑ | HMGCS2, OXTC1, CYP2E1, and SLC2A4 | Karjalainen et al. 2022 |
Dose-response chronic kidney disease (CKD) | Homoarginine ↑ | GATM (rs1145091) | Surendran et al. 2022 |
Waist circumference | Putrescine ↑ | AOC1 and JMJD1C | König et al. 2022 |
Coronary heart disease (CHD) | Octadecanedioate ↓ | CYP4F2 | Feofanova et al. 2020 |
Chronic kidney disease (CKD) | Lysine* ↑ | SLC7A9 (rs8101881) | Rueedi et al. 2014 |
Type 2 diabetes (T2DM) | Branched chain amino acids (BCAA) ↑ | PPM1K | Lotta et al. 2016 |
Coronary heart disease (CHD) & primary sclerosing cholangitis (PSC) | Leukotriene D4 ↓ | SLCO1B1 | Qin et al. 2020 |
Major adverse cardiovascular event (MACE) | 3-Indolepropionic acid (IPA) ↓ | ACSM5 and ACSM2B | Wang et al. 2021 |
Schizophrenia | N-delta-acetylornitine ↓ | NAT8 and SLC16A12 | Panyard et al. 2021 |
A new general strategy for analyzing genomic variance
Given the robust causal relationships found between certain clinical phenotypes, metabolites, and genetic variants, combining GWAS and metabolomics into mGWAS, followed by evaluation with Mendelian randomization, should become the new standard for identifying genomic variance and potential therapeutic targets. The key steps should include the following:
- In a population study with available genomic, metabolomic and longitudinal clinical information, select a phenotype of interest (e.g. myocardial infarction, type 2 diabetes, cancer).
- Conduct mGWAS by jointly evaluating GWAS and metabolomic data (especially metabolite ratios) to uncover highly relevant associations and/or new functional relationships to underlying genetic variance.
- Use Mendelian randomization to establish causality between genetic variant and phenotype. The shortlist of associations with proven causality can then be mined for feasible therapeutic drug targets.
- Repeat the Mendelian randomization with subgroups defined by specific longitudinal clinical information, such as drug exposure, to identify causal relationships between treatment and outcome (phenotype), and enable prediction of drug intervention effects.
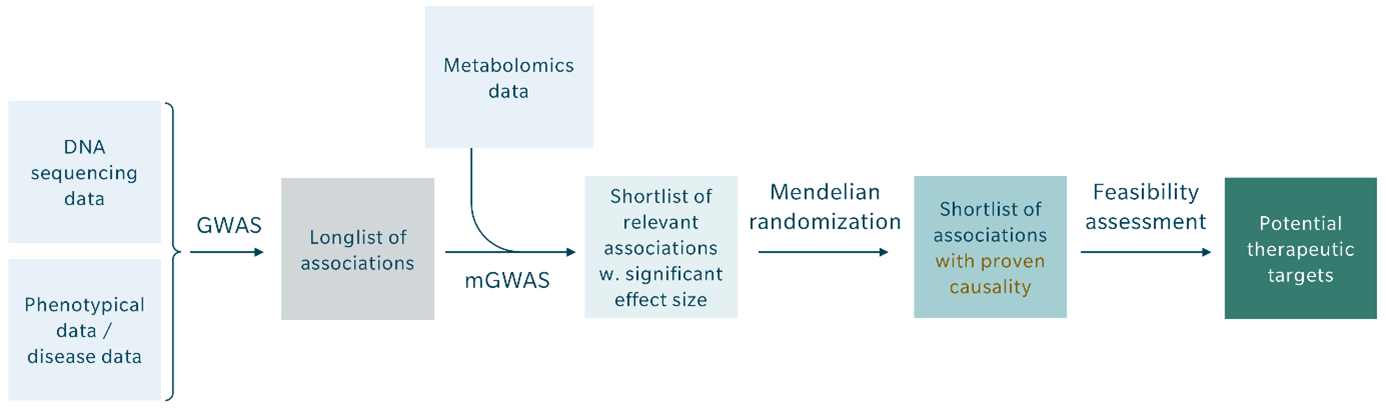
Where to start
Despite the undisputable advantages of combining GWAS with metabolomics and Mendelian randomization, it is still not a common approach. Among the more than 9000 GWAS that have been conducted, there are less than 70 mGWAS, and only about a dozen have incorporated Mendelian randomization, as shown in the table above. These numbers suggest that many existing GWAS could be combined with metabolomics to qualify results and derive causal links between gene variants and a clinical phenotype.
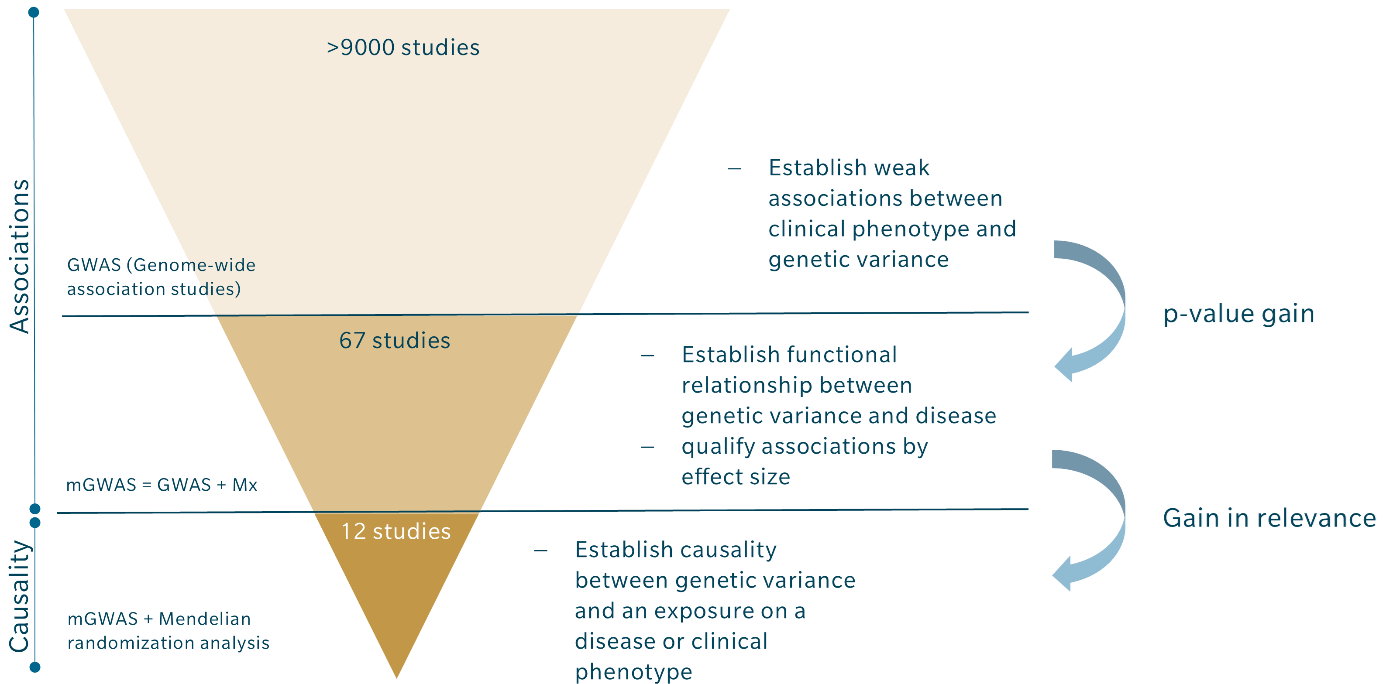
Many researchers performing GWAS may be unfamiliar with how to integrate metabolomics and conduct Mendelian randomization. To bring out this untapped potential, here are some helpful links:
- Prof. Karsten Suhre explains the principle behind mGWAS and Mendelian randomization very well in the book “Genetics meets metabolomics” (Genetics meets metabolomics 2012). For a preview, you can listen to Prof. Suhre talking about mGWAS and metabolite ratios in our podcast “The Metabolomist”.
- The mGWAS-Explorer lists details of 65 manually curated mGWAS studies, along with an mGWAS R package for download.
- biocrates’ multiomics data analysis service enables integration of metabolomics with genomics and other omic datasets.
With these resources, you should be able to use metabolomics to significantly improve fine-mapping of genomic data to phenotypes and identify causally supported phenotype-converting therapeutic targets.
Interested in conducting a broad metabolomics analysis to be integrated with your GWAS? Find out more about the biocrates MxP® Quant 500 XL kit.
References
Mountjoy, E. et al.: An open approach to systematically prioritize causal variants and genes at all published human GWAS trait-associated loci. (2021). Nat Genet | DOI: 10.1038/s41588-021-00945-5.
Sun, D. et al.: Why 90% of clinical drug development fails and how to improve it? 2022. Acta Pharm Sin B | DOI: 10.1016/j.apsb.2022.02.002.
Morgan, P. et al.: Impact of a five-dimensional framework on R&D productivity at AstraZeneca. 2018. Nat Rev Drug Discov | DOI: 10.1038/nrd.2017.244.
Petersen, A-K. et al.: On the hypothesis-free testing of metabolite ratios in genome-wide and metabolome-wide association studies. 2012. BMC Bioinformatics | DOI: 10.1186/1471-2105-13-120.
Montasser, ME. et al.: An Amish founder population reveals rare-population genetic determinants of the human lipidome. 2022. Commun Biol | DOI: 10.1038/s42003-022-03291-2.
Davies, NM. et al.: Reading Mendelian randomisation studies: a guide, glossary, and checklist for clinicians. 2018. BMJ | DOI: 10.1136/bmj.k601.
Genetics meets metabolomics: From experiment to systems biology. 2012. New York, Heidelberg: Springer | DOI: 10.1007/978-1-4614-1689-0.
Bowden, J. et al.: Meta-analysis and Mendelian randomization: A review. 2019. Res Synth Methods | DOI: 10.1002/jrsm.1346.
Chang, L. et al.: mGWAS-Explorer 2.0: Causal Analysis and Interpretation of Metabolite–Phenotype Associations. 2023. Metabolites | DOI: 10.3390/metabo13070826.
Yin, X. et al.: Genome-wide association studies of metabolites in Finnish men identify disease-relevant loci. 2022. Nat Commun | DOI: 10.1038/s41467-022-29143-5.
Karjalainen, MK. et al.: Genome-wide characterization of circulating metabolic biomarkers reveals substantial pleiotropy and novel disease pathways. 2022. medRxiv | DOI: 10.1101/2022.10.20.22281089.
Surendran, P. et al.: Rare and common genetic determinants of metabolic individuality and their effects on human health. 2022. Nat Med | DOI: 10.1038/s41591-022-02046-0.
König, E. et al.: Whole Exome Sequencing Enhanced Imputation Identifies 85 Metabolite Associations in the Alpine CHRIS Cohort. 2022. Metabolites | DOI: 10.3390/metabo12070604.
Feofanova, EV. et al.: A Genome-wide Association Study Discovers 46 Loci of the Human Metabolome in the Hispanic Community Health Study/Study of Latinos. 2020. Am J Hum Genet | DOI: 10.1016/j.ajhg.2020.09.003.
Rueedi, R. et al.: Genome-wide association study of metabolic traits reveals novel gene-metabolite-disease links. 2014. PLoS Genet | DOI: 10.1371/journal.pgen.1004132.
Lotta, LA. et al.: Genetic Predisposition to an Impaired Metabolism of the Branched-Chain Amino Acids and Risk of Type 2 Diabetes: A Mendelian Randomisation Analysis. 2016. PLoS Med | DOI: 10.1371/journal.pmed.1002179.
Qin, Y. et al.: Genome-wide association and Mendelian randomization analysis prioritizes bioactive metabolites with putative causal effects on common diseases. 2020. medRxiv | DOI: 10.1101/2020.08.01.20166413.
Wang, Z. et al.: Genome-wide association study of metabolites in patients with coronary artery disease identified novel metabolite quantitative trait loci. 2021. Clin Transl Med | DOI: 10.1002/ctm2.290.
Panyard, DJ. et al.: Cerebrospinal fluid metabolomics identifies 19 brain-related phenotype associations. 2021. Commun Biol | DOI: 10.1038/s42003-020-01583-z.